11 February 2022
h15-17 Greenwhich Mean Time / 16-18 CET
(check your local time here)
Abstract. Multi-layer artificial neural networks (ANNs) have been used to model many different neural and cognitive processes ranging from vision to memory to motor control. The recent resurgence of the use of these models in computational neuroscience stems from a rough similarity between artificial and biological neural networks and the ability to train these networks to perform complex high-dimensional tasks. Here I argue that in addition to models of specific neural computations, artificial neural networks should be used explicitly as a testing ground for the tools of systems neuroscience. Many tools commonly applied to neural data such as dimensionality reduction, representational similarity analyses, latent factor identification, and network analyses, can be validly applied to ANNs. ANNs are also fully observable and can be easily manipulated, meaning that any conclusions drawn from the application of these tools can be tested quickly in further `experiments’. Yet, due to their training, an algorithmic level of understanding of ANNs is not immediately available, making the use of analysis methods crucial for understanding them. Tools that successfully help us understand ANNs can be considered vetted for their use on real neural data, where testing of tools is slower and more difficult.
Grace Lindsay (University College London)
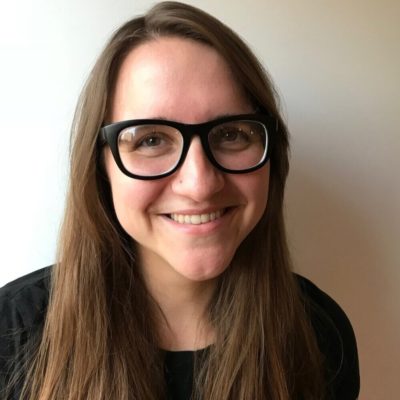
Practical information:
You can preview the paper here.
Join the meeting via webex (the link will be activated 10 mins before the talk.)
The next Neural Mechanisms Online webinar—”Testing the Tools of Systems Neuroscience on Artificial Neural Networks”—will be delivered by Grace Lindsay on Friday the 11th. See below for details about the free talk and how to join.
Testing the Tools of Systems Neuroscience on Artificial Neural Networks
Discussants
Rosa Cao (Stanford)
Matteo Colombo (Tilburg)