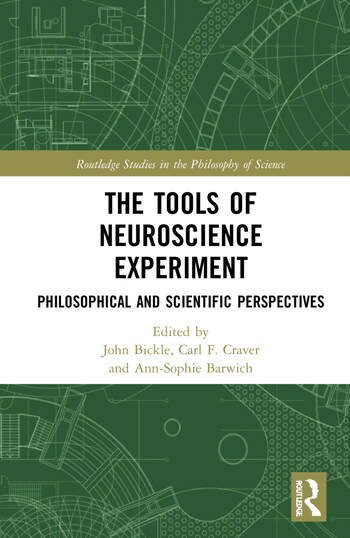
I use the recent emergence of optogenetics in neuroscience as an opportunity to explore how scientists evaluate their intervention tools. I look at how scientists promoted and defended optogenetics and what they took to constitute its improvement over time. My essay is guided by the aim of extracting a set of normative virtues for intervention, including: number, selectivity, and physiological relevance of variables targeted, the grain and range within which they can be manipulated, the valence and reversibility of the changes induced, and the efficacy, dominance, and determinism of the manipulation. Tool development often involves balancing these competing virtues when they trade off against one another. This list is thus usefully construed as an introductory step toward a virtue epistemology of intervention tools.
My paper focuses instead on tools for intervention. After all, experiments typically involve both intervening and detecting: ablations, electrodes, lasers and stains are as indispensable to scientific experiment as are detection apparatus (see Hacking 1983; Kästner 2017; Pearl 2009). My question is this: What makes one intervention tool better than another for extracting scientific knowledge from an experimental system?
Also of interest, I hope, is the mechanistic scaffolding that frames the discussion of those virtues, a scaffolding shared to some extent with Rick Shang’s (2021) insightful doctoral thesis on the evolution of PETT detection technology. Three core aspects of what might be called a mechanistic account of experimental tools are:
Shang, Rick. 2021. Positron Emission Tomography from 1930 to 1990: The Epistemology and Process of Scientific Instrumentation. PhD Dissertation; Washington University in St. Louis.
Kästner, Lena. 2017. Philosophy of Cognitive Neuroscience: Causal Explanations, Mechanisms and Experimental Manipulations. Walter de Gruyter GmbH & Co KG.
Weber, M. (2005) Philosophy of Experimental Biology. Cambridge University Press.
References
As Shang (2021) argues forcefully, niche construction can play a crucial role in this selective process. PET technology, as we now have it, emerged in the 1980s in part from a desire to find a marketable use for the short half-life radionuclides produced by Compton’s old scanner at Washington University. While PET scans delivered images with lower spatial resolution than those readily available through CT scans, the most obvious competitor in the imaging domain, the engineers and scientists working on PET exploited a feature of the materials at their disposal: time. Decay of radionuclides could be used to track even short time-frame physiological processes. The decision to chase time rather than space led to material changes to the design of the technology and the goals toward which it evolved.
In the case of optogenetics, this reveals an internal structure to the intervention process not recognized when interventions are thought of merely as singular exogenous causes. Entirely different parts of the optogenetic mechanism are responsible for determining what stimulus is delivered, how it is delivered, where it is delivered and when it is delivered. Because these parts of the optogenetic mechanism are modular, experimenters can swap out pieces, using this rather than that channel, expressing it in this location and not others, and expressing it at this time and not before or later. And because the system is modular in this sense, one is relatively safe in assuming that engineering adjustments in one part of the mechanism will not ramify to the others.
Why are scientific tools philosophically interesting? Because instruments are where the rubber of experimentation meets the road of world. In my paper, I explore the epistemic norms that govern when a tool can be used to coax useful information out of an experimental system. It is common to describe experimental tools as sensory prostheses. Telescopes, microscopes, thermometers and PETT scans show us things we cannot observe with our unaided senses. A natural philosophical project concerns how we know to trust what these detection systems tell us (see, e.g., Chang 2004; Staley 2020).
Philosophy and PNP Program
Feest, U. (2021) Data quality, experimental artifacts, and the reactivity of the psychological subject matter.European Journal for the Philosophy of Science. 12:13.
Shikore, J. 2019. The Structure and Function of Experimental Control in the Life Sciences. Philosophy of Science. 86: 203-218.
Washington University in St. Louis
Carl F. Craver
Staley, K. 2020. British Journal for the Philosophy of Science. Securing the Empirical Value of Measurement Results. 71:87-113.
Hacking, Ian, 1983. Representing and Intervening: Introductory Topics in the Philosophy of Natural Science. Cambridge University Press.
Pearl, Judea. 2009. Causality. Cambridge university press.
First, mechanisms. Intervention tools are mechanisms by which a researcher can alter some target feature or process. As designed mechanisms, they tend to be tidier than their “natural” counterparts¾ they tend to be modular, plug and play, readily decomposable into distinct mechanisms, which are themselves so decomposable. And each modular mechanism is often tasked with a specific function. Efforts to improve tools are thus simplified: one can tweak one module without changing how the other parts work. In other respects, tool improvement follows canalized paths enforced by basic decisions about how the mechanism should work.
Finally, artificial selection. Tools are adopted and retained when they contribute to their adopters’ epistemic aims. The norms governing that selection process for a particular tool are revealed explicitly or implicitly in the path of its evolution. Sometimes, tools evolve through intentional efforts to improve them, as when researchers made designer transgenic opsin molecules that could be stimulated at higher frequencies than could wild-type molecules without generating electrophysiological artifacts. Physiological relevance was a key selective force for optogenetics that is irreducible to and largely independent of, for example, concern with how surgical the intervention can be made.
Chang, H. 2004. Inventing temperature: Measurement and scientific progress. Oxford University Press.
Craver, C. F. And Dan-Cohen, T. forthcoming. Experimental artifacts. British Journal of Philosophy of Science
Sullivan, J. A. (2009). The multiplicity of experimental protocols: A challenge to reductionist and non-reductionist models of the unity of neuroscience. Synthese, 167(3), 511–539.
Despite numerous positive inroads (e.g., Chang 2004; Craver and Cohen forthcoming; Feest 2021; Sullivan 2009; Staley 2020; Schickore 2019; Shang 2021; Weber 2004; and this exciting volume as a whole) the philosophy of science has yet to deliver anything resembling a general philosophical orientation to the topic of experimentation. As such, our understanding of how representing and intervening contribute to the production of knowledge remains to be written. My paper focuses on the epistemology of intervention tools, on what it means to make an intervention tool better for doing science. And in the process, I sketch a materially-grounded and mechanistic approach to addressing that question.
Lewis, David. 1979. “Counterfactual Dependence and Time’s Arrow.” Noûs 13 (4): 455–76. https://doi.org/10.2307/2215339.
Woodward, James. 2003. Making Things Happen: A Theory of Causal Explanation. Oxford University Press.
Second, satisficing. No real interventions are ideal. Ideal interventions (in Woodward’s sense) are frequently impossible in practice: we don’t have the tools to effect them, they are dangerous, they are simply physically impossible (e.g., a miracle violation of conservation), or they are impractical. So science is typically fumbling along with interventions somewhere in the range between the ideal and the hopeless. More fundamentally, Woodward’s notion of an ideal intervention emphasizes only one normative dimension of intervention tools (precision) among the ten I discuss. Real science, as opposed to the science of our philosophic imaginations, involves learning to get (more and more) useful signals with invariably disappointing tools.
Craver, Carl F., and Lindley Darden. 2013. In Search of Mechanisms: Discoveries across the Life Sciences. University of Chicago Press.