Abstract. Information that is shared across brains is encoded in idiosyncratic fine-scale functional topographies. Hyperalignment captures shared information by projecting pattern vectors for neural responses and connectivities into a common, high-dimensional information space, rather than by aligning topographies in a canonical anatomical space. Individual transformation matrices project information from individual anatomical spaces into the common model information space, preserving the geometry of pairwise dissimilarities between pattern vectors, and model cortical topography as mixtures of overlapping, individual-specific topographic basis functions, rather than as contiguous functional areas. The fundamental property of brain function that is preserved across brains is information content, rather than the functional properties of local features that support that content. In this Perspective, we present the conceptual framework that motivates hyperalignment, its computational underpinnings for joint modeling of a common information space and idiosyncratic cortical topographies, and discuss implications for understanding the structure of cortical functional architecture. Hyperalignment:
Modeling shared information encoded in idiosyncratic cortical topographies
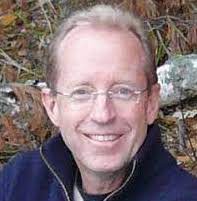
James V. Haxby (Dartmouth College) Videos of prior talks can be found on the Neural Mechanisms YouTube channel.
Join the meeting via Webex (the link will be activated 10 mins before the talk) |
h 14-16 Greenwhich Mean Time / 16-18 CEST (check your local time here)
You can find the paper here.
The next Neural Mechanisms Online webinar “Hyperalignment: Modeling shared information encoded in idiosyncratic cortical topographies” will be delivered by James V. Haxby on June 3rd. See below for details about the free talk and how to join. Practical information: